Election Forecasting
Where Art Meets Science: A Deep Dive into Methodologies
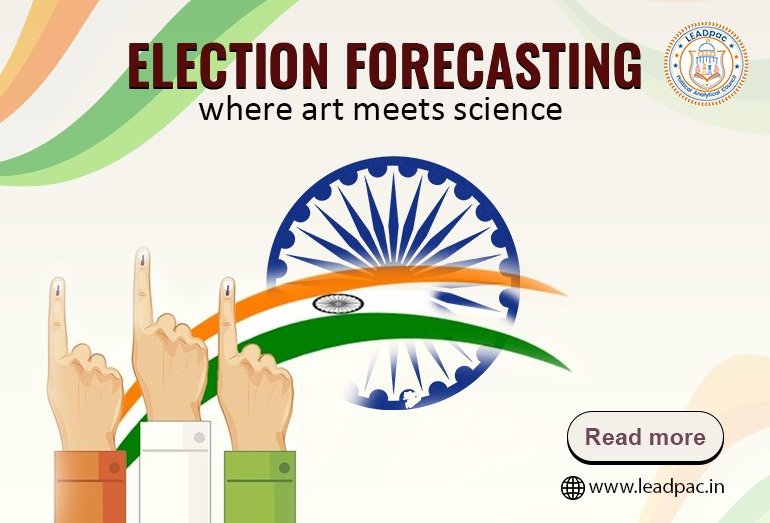
Election Forecasting stands at the intersection of data analysis, historical context, and predictive Modeling. In this blog, we will unravel the intricate methodologies employed in predicting election outcomes, shedding light on the pivotal role of data, historical trends, and sophisticated Modeling techniques.
1. Data Collection:
– The foundation of Election Forecasting lies in robust data collection. Analysts gather a wide array of data points, including voter demographics, past election results, economic indicators, and polling data.
– Polling data is particularly crucial, offering insights into voter preferences, candidate popularity, and potential shifts in public sentiment leading up to the election.
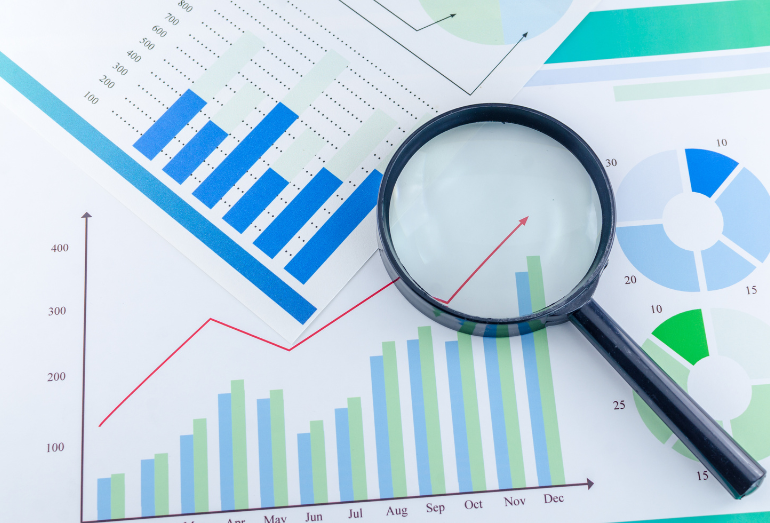
2. Historical Trends Analysis:
– Understanding Historical Voting Patterns is essential for Forecasting future Elections. Analysts delve into past election results at various levels (national, state, local) to identify trends, swing regions, and demographic shifts.
– By analysing Historical Data, forecasters can uncover correlations between different variables and use this information to refine their predictive models.
3. Modeling Techniques:
– Election Forecasters employ a diverse set of Modeling Techniques to predict outcomes with varying degrees of accuracy. Common approaches include statistical Modeling, machine learning algorithms, and simulation methods.
– Statistical Models, such as regression analysis, help quantify the relationship between variables and make predictions based on historical data.
– Machine Learning Algorithms, like neural networks or random forests, can process vast amounts of data to identify complex patterns and make more nuanced predictions.
– Simulation Methods simulate thousands of election scenarios to assess the likelihood of different outcomes, providing a probabilistic view of election results.
4. Poll Aggregation and Weighting:
– Poll Aggregation involves combining multiple polls to create a more comprehensive view of voter preferences. Analysts must carefully weigh each poll based on factors like sample size, methodology, and historical accuracy.
– Weighting is crucial to ensure that polls with higher credibility and accuracy contribute more significantly to the forecast, minimizing the impact of outliers or biased polls.
5. Uncertainty and Margin of Error:
– Election Forecasting inherently involves uncertainty. Forecasters often provide a margin of error to indicate the range within which the actual election outcome is likely to fall.
– Factors like undecided voters, last-minute shifts, and unforeseen events can introduce volatility and widen the margin of error in election predictions.
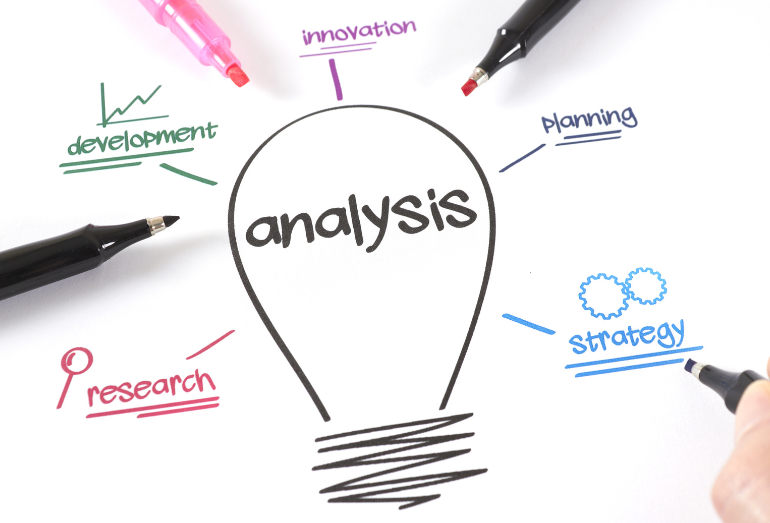
6. Sentiment Analysis:
– In addition to traditional polling data, Sentiment Analysis has emerged as a powerful tool for understanding public opinion. This technique involves mining social media, news articles, and other online sources to gauge the mood and sentiments of voters.
– By analyzing the language used in online conversations, Sentiment Analysis can uncover subtle shifts in public perception towards candidates, parties, or key issues, providing valuable insights for election forecasters.
7. Geospatial Analysis:
– Geospatial Analysis plays a crucial role in understanding regional variations in voting behavior. By mapping demographic data, economic indicators, and historical voting patterns onto geographic regions, analysts can identify key battlegrounds and potential swing states.
– This spatial perspective allows forecasters to tailor their predictions to specific regions, accounting for localized factors that may influence electoral outcomes, such as demographic shifts, economic conditions, or campaign strategies.
8. Ensemble Modeling:
– Ensemble Modeling combines multiple forecasting techniques to improve prediction accuracy. Instead of relying on a single model, ensemble methods integrate the forecasts generated by various models to produce a more robust and reliable prediction.
– By leveraging the strengths of different modeling approaches, such as statistical models, machine learning algorithms, and simulation methods, ensemble modeling can mitigate the weaknesses of individual models and provide more accurate forecasts, especially in complex and dynamic electoral environments.
9. Dynamic Updating:
– Election Forecasting is an iterative process that requires constant monitoring and updating as new data becomes available and the electoral landscape evolves. Forecasters employ dynamic updating techniques to incorporate real-time information and adjust their predictions accordingly.
– By continuously refining their models based on the latest data and trends, forecasters can provide timely and accurate predictions that reflect the most current state of the race, enhancing the usefulness of their forecasts for decision-makers, policymakers, and the general public.
10. Scenario Analysis:
– In addition to predicting the most likely outcome, Election Forecasters often conduct scenario analysis to assess the potential impact of various events or contingencies on the election result. This involves simulating different scenarios, such as changes in voter turnout, shifts in support among demographic groups, or unexpected external events, to evaluate their potential effects on the electoral outcome.
– Scenario Analysis helps stakeholders anticipate and prepare for potential risks and uncertainties, providing valuable insights into the resilience of electoral systems and the dynamics of political processes in the face of uncertainty and change.
Conclusion:
Election Forecasting is a multidimensional process that blends data analysis, historical insights, and sophisticated modeling techniques to predict electoral outcomes. By leveraging a combination of data-driven approaches, contextual understanding, and embracing advanced methodologies, forecasters strive to offer valuable insights into the complex dynamics of democratic processes. Through these efforts, they navigate the complexities of modern elections with greater precision and insight, enriching our understanding of democratic processes and helping to inform informed decision-making in an increasingly complex and dynamic political landscape.